Studio Ghibli’s aura, Gemini’s takeover, and opening the black box
Currents: AI & Energy Insights - March 2025
Welcome back to Currents, a monthly column from Reimagine Energy dedicated to the latest news at the intersection of AI & Energy. At the end of each month, I send out an expert-curated summary of the most relevant updates from the sector. The focus is on major industry news, published scientific articles, a recap of the month’s posts from Reimagine Energy, and a dedicated job board.
1. Industry news
A breakthrough in image generation
OpenAI released a new image generation model and announced gaining 1 million new users in one hour thanks to it. I wrote a thorough analysis of the models’ capabilities (and shortcomings) in my latest article. Give it a read if you haven’t already:
I tested GPT-4o image generation on 5 energy-sector use cases
GPT-4o image generation was released last week and quickly went viral, thanks to its ability to accurately edit existing images and replicate artistic style, including Studio Ghibli’s signature aesthetic.
What I’m thinking
One of the most impressive features of the new OpenAI image generation model is its ability to accurately edit an image following any artistic style. As the internet quickly realized this, images generated with the signature aesthetic from Japanese film animation studio Studio Ghibli went viral. While the model’s ability to replicate Studio Ghibli’s style is impressive (and entertaining), I also have significant concerns:
The appropriation of vast amounts of artists' work scraped from the internet without consent, credit, or compensation by large AI corporations seems ethically dubious at best.
The beauty of something like the Ghibli aesthetic isn’t just about the visual output. It is the meticulous craftsmanship, the human dedication, the process and detail involved. German philosopher Walter Benjamin writes in his essay The Work of Art in the Age of Mechanical Reproduction about the “aura” of an artwork, and how that is linked to its uniqueness and authenticity. I fear that the ability to mass produce any artistic style, aesthetic or imagery will contribute to the de-evaluation of such pieces.
How much electricity for compute, and water for data center cooling, did it take to “Ghiblify” the internet? OpenAI doesn’t share any kind of data about this. We need to demand more transparency from LLM companies so that we can better decide which models to use and how frequently.
Gemini takes the lead
While OpenAI topped all the headlines because of its new image generation model, Google quietly took the lead in the “smartest model” race with Gemini 2.5.
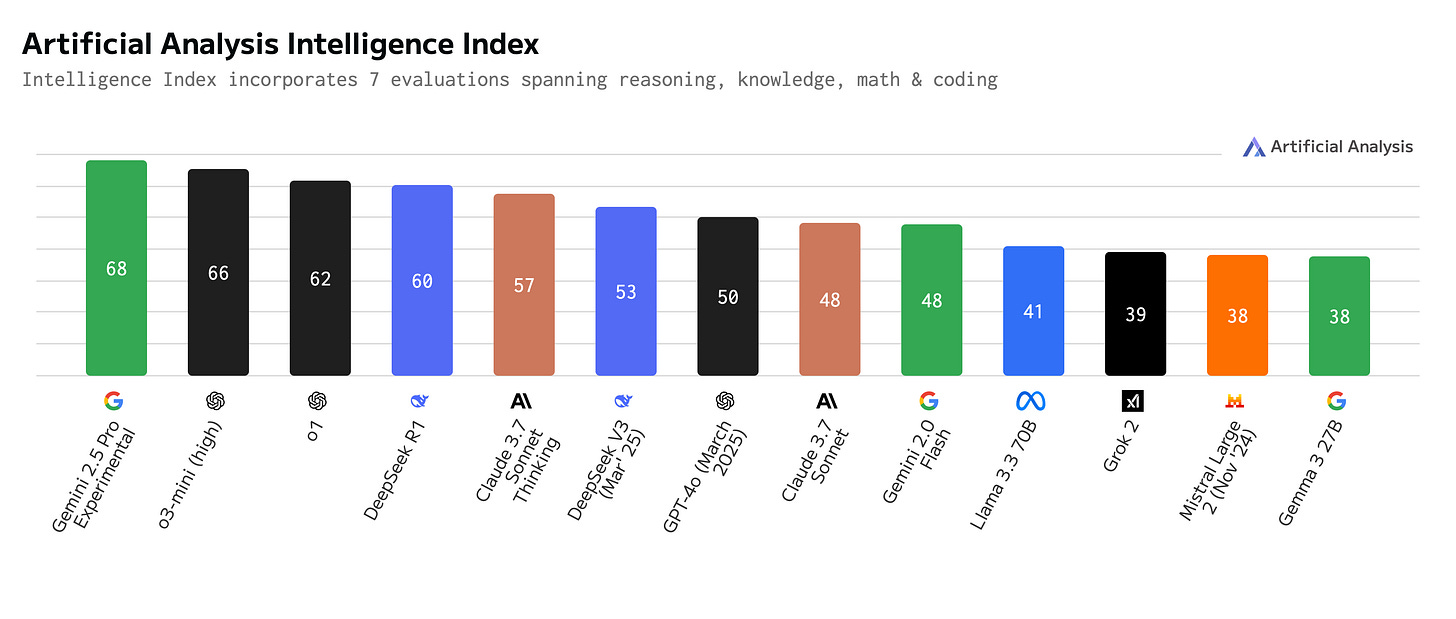
If you’re curious about the latest updates on which models are best at what, this is a good overview: Claude is still the best at coding, but Gemini 2.5 now leads in almost all other areas. OpenAI o1 is still the best for multilingual tasks, closely followed by Llama 70B and Mistral.
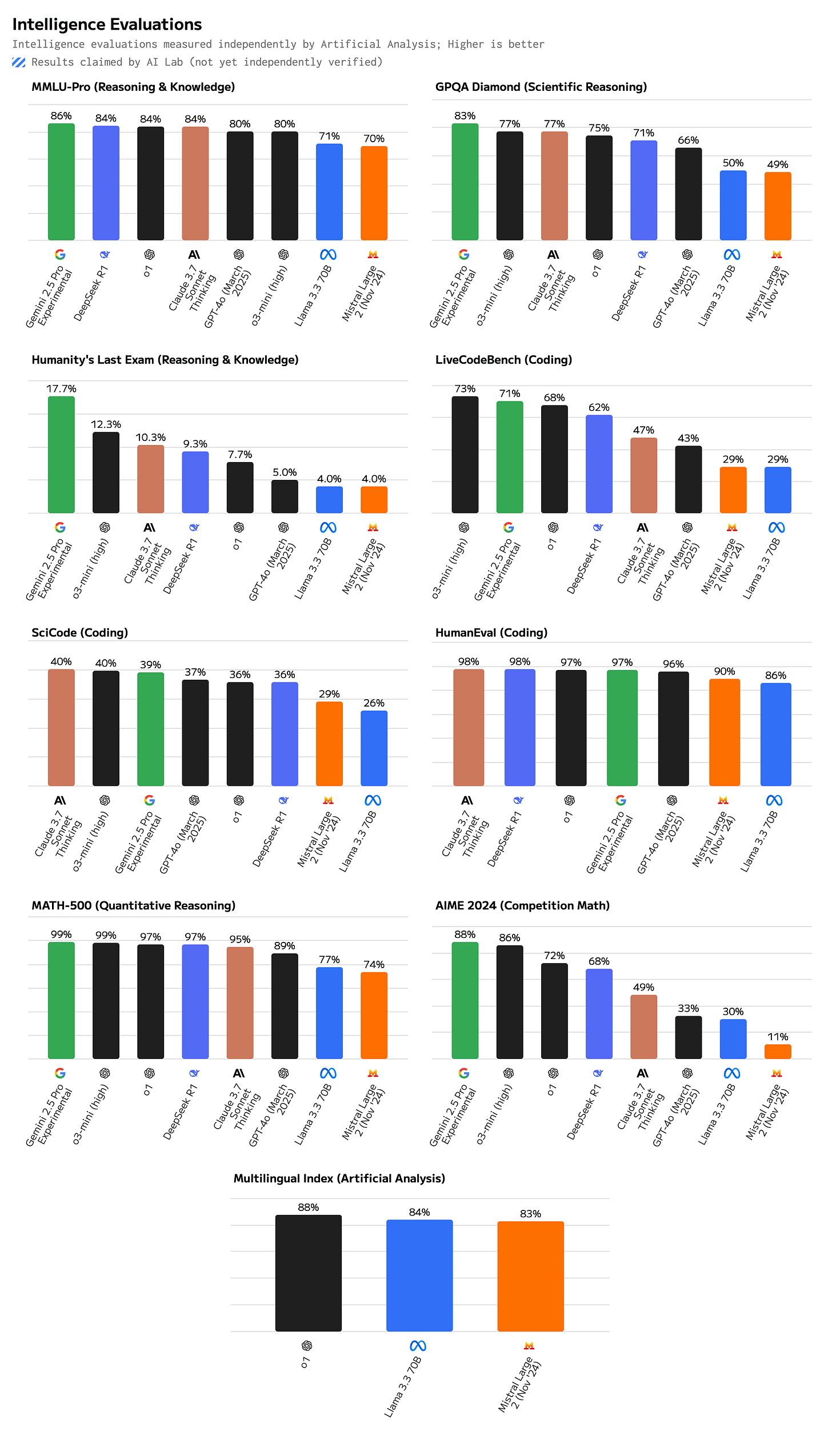
What I’m thinking
If you haven’t tested a reasoning model yet, I suggest playing around with Gemini 2.5 on Google AI Studio (it’s free for now), to experience the current capabilities of best-in-class LLMs.
The API pricing for Gemini 2.5 is still unknown, but considering Gemini 2.0 has one of the lowest price/token rates (30 times lower than GPT-4o), there may soon be little reason to use OpenAI’s API unless they release a significantly better model.
Model Context Protocol: extending AI’s reach into the physical and operational world
One trending concept this past month was the Model Context Protocol (MCP), created and open-sourced by Anthropic late last year. MCP’s core purpose is to act as a standard communication layer between AI models and external data/tools. It defines how an AI agent can query or interact with external systems in a uniform way. A popular analogy is that MCP is like a “USB-C port” for AI: a single, standardized connector that works across many types of devices (in this case, data sources, tools, and applications).
In the buildings sector, MCP could serve as the connection between an AI assistant and a building’s automation system. By deploying MCP servers that interface with IoT devices, an AI agent can manage the environment through natural language commands or autonomous policies. EMQ, a software provider for open-source IoT data infrastructure, just published an overview of potential solutions to use MCP in combination with MQTT, another messaging protocol designed for machine-to-machine communication. One example from their blog would be a scenario in which a user could say, “I’ll be home in an hour, set the living room temperature to 25°C and humidity to 40%,” and behind the scenes an AI using MCP would communicate with the thermostat and humidifier to carry that out.
Home Assistant, a popular open-source home automation software, already added MCP support in their February update. If you're using it, you can test MCP now.
What I’m thinking
MCP extends AI’s reach into the physical and operational world, making it highly relevant for energy and buildings. In a demand-side flexibility scenario, for instance, an AI agent might react to certain signals by temporarily reducing HVAC usage during grid stress. These complex cross-system optimizations become more feasible when an AI can communicate with all necessary subsystems via one protocol.
One concern from my side: we’re increasingly enabling AI agents to operate autonomously in the world, which is good… until it isn’t. This doesn’t mean we shouldn't develop this technology, but we must concurrently research and invest in AI safety, build guardrails, and remain mindful of potential misuse.
2. Scientific publications
Tracing the thoughts of large language models
Two interesting research papers from Anthropic were released this past month. Auditing Language Models for Hidden Objectives, investigates the feasibility of detecting hidden undesirable goals within models. Researchers trained a model with a hidden objective and conducted blind auditing games to test detection methods. Results showed that teams using interpretability tools, behavioral attacks, and training data analysis could successfully uncover the hidden objective, while teams limited to black-box interaction (API access) could not. The second paper, On the Biology of a Large Language Model, explores the internal computational mechanisms of Claude Haiku, employing circuit tracing to reverse-engineer how the model performs tasks such as reasoning, planning, and multilingual processing. Intuitively, this was achieved by replacing the model's complex neurons with simpler, understandable "features" and mapping the flow of information between them.
What I’m thinking
The idea of LLMs being used by governments and corporations to control and influence how people think is deeply concerning. xAI’s Grok, for instance, has already been called out for sharing disinformation or censoring criticism of Elon Musk and Donald Trump. Within this context, being able to investigate potential biases maliciously introduced in LLMs seems very relevant.
Another interesting point is that the circuit tracing methods introduced in the second paper might be used to improve the audits discussed in the first. Instead of relying solely on finding suspicious training data or specific behavioral failures, auditors could potentially use circuit analysis to directly inspect for known problematic mechanisms or to understand the root cause of a behavior flagged during an audit.
While no company is “perfect”, Anthropic is among the few dedicating resources to interpretability and safety research, I hope they maintain this focus!
Generating synthetic fault data with GANs
Hybrid-CGAN: A Hybrid approach combining simulation and generative adversarial networks for fault detection and diagnosis in buildings. In this paper, authors from the National University of Singapore propose combining Generative Adversarial Networks (GANs) with simulation data from EnergyPlus to generate realistic synthetic fault data, reducing the need for actual, hard-to-get fault samples. The study focuses on faults within an Air Handling Unit (AHU) system, representative of a large office building. Results showed synthetic data generated in this way is significantly more realistic than simulation data alone.
What I’m thinking
Fault detection data is very hard to retrieve and typically locked in proprietary BMS systems. We previously discussed how data scarcity is a significant issue in the sector and how transfer learning is one of the potential solutions. Generating synthetic data is yet another solution to this problem and a promising one for certain applications. The authors mention that the data will be made available on request. It would be great if they could open-source both code and data, so that the community could validate and advance this very relevant research!
3. Reimagine Energy publications
I already mentioned at the beginning of this issue, this month’s post is an accessible read that you can share with all your energy-loving friends and colleagues:
I tested GPT-4o image generation on 5 energy-sector use cases
GPT-4o image generation was released last week and quickly went viral, thanks to its ability to accurately edit existing images and replicate artistic style, including Studio Ghibli’s signature aesthetic.
4. AI in Energy job board
This space is dedicated to job posts in the sector that caught my attention during the last month. I have no affiliation with any of them, I’m just looking to help readers connect with relevant jobs in the market.
Data Scientist & Building Energy Specialist at Open Technologies
Lead Data Scientist for DER & Policy Forecasting at National Grid
AI Controls Engineer – HVAC Automation at Brainbox AI
PhD scholarship in Impact and Scalability of Advanced Controls for Energy Demand Flexibility in Buildings at DTU - Technical University of Denmark
Conclusion
With so much going on in the sector, it’s not easy to follow everything. If you’re aware of anything that seems relevant and should be included in Currents (job posts, scientific articles, relevant industry events, etc.), please reply to this email or reach out on LinkedIn and I’ll be happy to consider them for inclusion.
💡